support@comsol.com
Uncertainty Quantification Updates
For users of the Uncertainty Quantification Module, COMSOL Multiphysics® version 6.1 introduces the ability to use experimental data for calibrating input uncertainty and new ways for specifying input parameters. Learn more about these updates below.
Inverse Uncertainty Quantification
You can now use experimental data to calibrate the unknown probability distributions of input parameters. The new Inverse Uncertainty Quantification study type is used to compute the posterior distribution of the input parameters — that is, the calibration parameters that best reflect their distribution based on the experimental data and prior knowledge of the calibration parameters. The Markov chain Monte Carlo (MCMC) method is used to infer the posterior distribution of the calibration parameters by constructing Markov chains whose stationary distribution is the posterior distribution. This study type propagates the experimental data backward to gain knowledge of the calibration parameters. To gain knowledge of the quantities of interest, you can compare this study type with the existing Uncertainty Propagation study type, which propagates a known parameter's distribution forward.
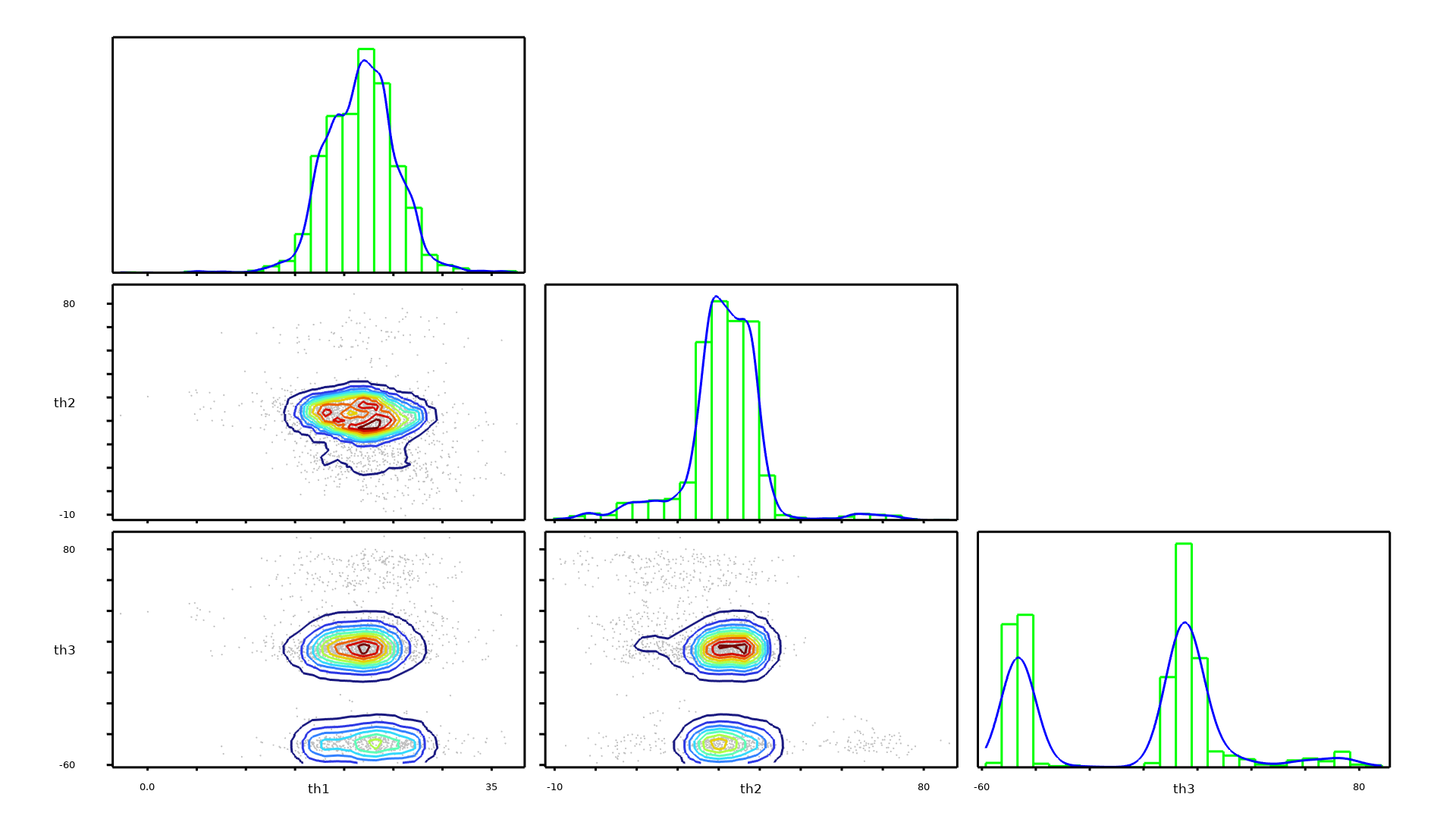
Additional Schemes for Input Parameters
There are new ways to specify input parameters, parameters used for surrogate-based Monte Carlo analysis, and parameters used for surrogate model verification. The parameters can take their values from analytic distributions, data columns from results tables, or specified values.